Introduction to Learning Analytics and Predictive Modeling
Unveiling the Power of Data: Exploring Learning Analytics
Have you ever wondered how educators can leverage mountains of data to gain valuable insights into student performance and improve educational outcomes? Welcome to the world of learning analytics! Learning analytics is the process of collecting, analyzing, and interpreting vast amounts of data generated by students’ educational activities.
This powerful tool aims to enhance teaching and learning experiences by uncovering patterns, identifying trends, and providing actionable information that can transform education as we know it. At its core, learning analytics seeks to make sense of the digital footprints left behind by students as they navigate through various learning environments.
By harnessing this wealth of information, educators can gain a deeper understanding of how learners engage with course materials, identify areas where students struggle or excel, and ultimately tailor their instructional approaches accordingly. But learning analytics is not just limited to retrospective analysis; it also enables a proactive approach through predictive modeling.
Predictive Modeling: Predicting Success Before It Happens
Imagine having the ability to predict which students are most likely to face obstacles on their academic journey even before they encounter them. That’s where predictive modeling comes into play in education. Predictive modeling utilizes statistical algorithms and machine learning techniques to mine past data patterns and make informed predictions about future outcomes.
In the realm of education, this groundbreaking approach allows us not only to anticipate potential pitfalls but also intervene early enough to prevent them from derailing student success. By leveraging diverse sources such as demographic information, academic records, assessment scores, or even social interactions within online learning platforms, predictive models employ sophisticated algorithms that identify risk factors associated with student underperformance or disengagement.
These models provide educators with invaluable insights into which students may require additional guidance or support during their educational journey. The importance of proactive identification cannot be overstated, as swift intervention can significantly boost outcomes for at-risk students and increase the likelihood of their academic achievements.
The Urgency to Identify At-Risk Students Early On
In an ideal world, no student would ever slip through the cracks, but we know that’s not always the case. Identifying at-risk students early on is of paramount importance to ensure that no child falls behind or fails to reach their full potential. Early identification allows educators to pinpoint students who may be struggling academically, socially, or emotionally before these challenges escalate and become more difficult to address.
By employing learning analytics and predictive modeling techniques, educational institutions can take a proactive stance in supporting vulnerable students. When we identify at-risk individuals early on, we afford them a greater chance of success by providing timely interventions and tailored support systems.
This approach not only empowers educators to personalize their teaching strategies but also encourages a nurturing environment where each student’s unique needs are acknowledged and addressed. So buckle up as we dive into the fascinating realm of learning analytics and predictive modeling in education – an innovative landscape brimming with possibilities to revolutionize how we educate our future generations!
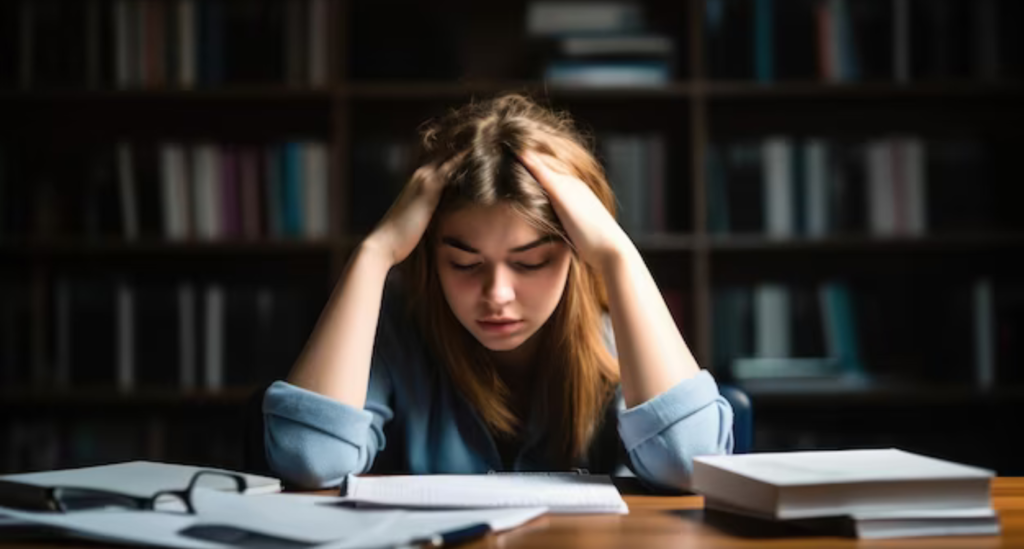
Understanding At-Risk Students
Defining the At-Risk Student: Navigating Troubled Waters
When it comes to understanding at-risk students, it’s crucial to first define who they are and what sets them apart from their peers. At-risk students are individuals who face a higher probability of experiencing difficulties in their educational journey due to a variety of factors.
These factors can encompass both internal and external challenges that hinder their academic progress and overall success. Characteristics commonly associated with at-risk students include poor attendance, below-average grades, low motivation or engagement, behavioral issues, or even mental health concerns.
Additionally, some may come from disadvantaged backgrounds where socioeconomic disadvantages pose significant hurdles to overcome. It is important to recognize that being an at-risk student does not imply incompetence or a lack of potential; rather, it signifies a need for extra support and attention in order to thrive academically.
Factors Unraveled: Peering into the Complexity
The factors contributing to student at-riskness are multi-faceted and intertwined. One prominent influence is a student’s socio-economic background. Economic instability or limited access to resources can create barriers that impede learning opportunities.
For instance, financial difficulties might restrict access to supplementary educational materials or limit participation in extracurricular activities crucial for holistic development. Another critical factor is prior academic performance.
Students who have consistently struggled academically may face challenges in keeping up with the curriculum and building foundational skills necessary for future success. Additionally, personal circumstances such as family dynamics or health issues can significantly impact a student’s ability to concentrate on their studies.
The Early Bird Catches Success: Impact of Early Identification
Early identification of at-risk students plays a pivotal role in shaping positive outcomes for their academic journey. When these students are identified early on through learning analytics and predictive modeling techniques, educators and institutions can intervene proactively.
By targeting the root causes of their struggles, educators can provide tailored support and interventions that address specific needs. Through timely interventions and personalized strategies, the impact of early identification positively influences student success.
Not only does it increase the chances of at-risk students catching up with their peers academically, but it also bolsters their confidence and motivation to persevere. Early intervention allows for a more targeted approach towards building foundational skills, addressing behavioral concerns, and fostering a supportive learning environment that paves the way for long-term achievement.
Understanding at-risk students requires acknowledging their unique characteristics and recognizing the diverse factors that contribute to their at-riskness. By identifying these individuals early on in their academic journey, educators can provide them with the specialized support they need to overcome challenges and pursue success.
Data Collection for Learning Analytics
Sources of data (e.g., student information systems, learning management systems)
Data collection lies at the heart of effective learning analytics. Various sources provide valuable insights into student behavior and performance. Student information systems (SIS) serve as a treasure trove of administrative data, housing vital details like enrollment records, attendance, and grades.
These systems offer a comprehensive view of each student’s academic journey, making them an essential source for predictive modeling. In addition to SIS, learning management systems (LMS) also play a crucial role in data collection.
LMS platforms record students’ interactions within online courses, capturing valuable information such as time spent on assignments, quiz scores, and forum participation. By integrating LMS-generated data with other sources like SIS or even social media platforms where students engage in educational discussions outside the classroom, educators gain a more holistic understanding of their students’ behavior and engagement patterns.
Types of data collected (e.g., demographic, academic, behavioral)
Learning analytics thrives on diverse datasets that encompass various dimensions of student life. Demographic data provides insights into factors such as age, gender, ethnicity, and socio-economic background—information that helps identify potential discrepancies in educational outcomes across different groups. Academic data includes prior achievement records like test scores or GPA that aid in establishing baselines for performance comparisons.
Furthermore, behavioral data plays a critical role in identifying at-risk students early on. It encompasses online interactions within the LMS platform (e.g., frequency of logins or participation in discussions), attendance records both online and offline if applicable to the learning environment (e.g., physical classes), engagement with course materials (e.g., video views or time spent), and even extracurricular activities linked to the institution.
Challenges in Data Collection and Privacy Concerns
While the potential benefits of data collection for learning analytics are immense, there are significant challenges associated with it. One key hurdle is the fragmentation and siloing of data across different systems.
Integrating data from disparate sources like SIS, LMS, and external platforms often requires substantial effort in terms of system integration and data cleaning. Moreover, privacy concerns surrounding student data cannot be overlooked.
Safeguarding sensitive information is paramount to ensure ethical use of learning analytics. Organizations must adhere to strict privacy policies that ensure student confidentiality, consent for data usage, and comply with relevant regulations such as the Family Educational Rights and Privacy Act (FERPA) in the United States.
Finding the right balance between collecting pertinent information for predictive modeling while respecting students’ privacy rights is a continuous challenge in this field. Striking the right balance enables educational institutions to leverage learning analytics effectively while maintaining trust among students and stakeholders alike.
Analyzing Data Using Predictive Models
Overview of Predictive Modeling Techniques: Unleashing the Power of Machine Learning Algorithms
When it comes to analyzing data and predicting outcomes, machine learning algorithms steal the spotlight. These algorithms, embedded within predictive models, have the ability to learn from historical data and make intelligent predictions about future events.
With a multitude of machine learning techniques available, such as decision trees, neural networks, and support vector machines, educators can harness this power to identify at-risk students early on. Decision trees are like a flowchart that breaks down data into smaller subsets based on various attributes.
It follows a series of if-then rules to predict outcomes. Neural networks mimic the human brain by processing information through interconnected layers of nodes called neurons.
They can detect intricate patterns in complex datasets that might go unnoticed otherwise. Support vector machines excel at classifying data into different categories by creating boundaries between them in high-dimensional space.
Preprocessing Steps: Cleaning Data and Engineering Features for Optimal Results
Before jumping into predictive modeling, one must undertake crucial preprocessing steps to ensure accurate insights. Data cleaning involves removing any inconsistencies or errors in the dataset that may affect its integrity and reliability.
This includes handling missing values, dealing with outliers, and resolving inconsistencies. Feature engineering is another critical aspect where domain knowledge shines through.
By carefully selecting relevant features, educators can provide models with valuable insights about student performance indicators such as attendance records, grades from previous semesters, or engagement level with online learning platforms. Transforming raw data into meaningful features allows models to capture relevant patterns and make accurate predictions.
Model Selection and Evaluation Metrics: Finding the Right Fit for Accuracy
Selecting an appropriate model is like choosing the right tool for the job at hand—a crucial step to achieve accurate results in predictive modeling endeavors. Educators must consider the specific problem they want to address, the available data, and the complexity of the learning analytics task. Once a model has been selected, it is essential to evaluate its performance.
Various evaluation metrics come into play, such as accuracy, precision, recall, and F1 score. Accuracy measures how often the model predicts correctly.
Precision captures the proportion of true positive predictions out of all positive predictions made by the model. Recall assesses how well a model identifies true positives out of all actual positive cases.
By carefully selecting an appropriate machine learning technique, preparing data through cleaning and feature engineering, and evaluating models using relevant metrics, educators can unlock valuable insights from their predictive models. Harnessing these techniques enables early identification of at-risk students and empowers educators to intervene promptly with targeted support mechanisms for improved student outcomes.
Identifying At-Risk Students Early On
Defining Indicators or Risk Factors for Early Identification: Decoding the Signs
When it comes to identifying at-risk students, it’s essential to understand the indicators or risk factors that can help us spot them before they fall through the cracks. One crucial element is attendance. Poor attendance might seem like a harmless hiccup, but it can be a telling sign of disengagement or external challenges that students are facing.
Course engagement is another critical factor to consider. It goes beyond merely attending classes; we need to delve into how students actively participate and interact with course materials and activities.
Are they consistently completing assignments? Do they engage in discussions, ask questions, or seek clarification?
Monitoring these behaviors can give us valuable insights into their level of involvement and motivation. Moreover, academic performance history plays a crucial role in identifying at-risk students early on.
By analyzing past grades and assessments, we can uncover patterns that may indicate struggles in specific subjects or a general downward trajectory. These indicators act as red flags that guide us towards implementing targeted interventions to address each student’s unique needs.
Building Predictive Models: Unleashing the Potential of Historical Data
Harnessing the power of historical data is where predictive modeling shines bright. By extracting meaningful patterns from this data treasure trove, we can develop models that accurately predict which students are most at risk of falling behind academically.
Predictive models typically utilize machine learning algorithms that analyze various factors simultaneously – from demographic information to previous academic achievements and even socio-economic background. These algorithms learn from historical data patterns and make precise predictions about individual student success trajectories.
By training these models on vast datasets encompassing diverse student profiles, we ensure their accuracy when applied to real-time scenarios. The more comprehensive the historical data available for analysis, the more refined our predictions become.
Implementing Real-time Monitoring Systems: The Power of Swift Intervention
To truly make a difference in the lives of at-risk students, we must implement real-time monitoring systems that enable continuous assessment. Waiting until the end of the semester to uncover academic struggles is far from ideal – we need to intervene as soon as warning signs emerge.
Using advanced technologies and learning management systems, educators can track attendance and course engagement in real-time. This allows for immediate identification of students who might be veering off-track.
By acting swiftly, teachers can establish a proactive dialogue and offer personalized support, ensuring that struggling students receive timely interventions tailored to their needs. Furthermore, real-time monitoring systems provide educators with valuable insights into student progress throughout the semester.
Continuous assessment enables ongoing adjustments to instructional strategies and interventions based on up-to-date information. This dynamic approach ensures that support is not only provided when it’s needed but is also constantly adapted to meet evolving student needs.
By combining defined indicators for early identification with predictive models built on historical data analysis and implementing real-time monitoring systems, we create an ecosystem that empowers educators to intervene promptly and effectively cater to at-risk students’ needs. Through these efforts, we pave the way for increased success rates among vulnerable learners while nurturing an educational environment where no one is left behind.
Providing Support to At-Risk Students
Interventions based on identified risk factors (e.g., personalized tutoring, mentoring programs)
One effective intervention strategy is personalized tutoring, where experienced educators tailor their teaching methods to meet the unique needs of each student. By identifying specific areas of struggle and targeting them with customized lessons and exercises, tutors can provide the targeted support necessary for these students to thrive academically.
Additionally, mentoring programs can play a vital role in supporting at-risk students. Pairing these students with mentors who have overcome similar challenges fosters a sense of connection and guidance.
Through regular meetings and open communication channels, mentors can offer guidance not only in academics but also in personal growth and development. This mentor-student relationship helps build resilience, confidence, and motivation that may have otherwise been difficult for at-risk students to cultivate on their own.
Effective communication strategies with students, parents, and teachers
Subtitle: Bridging the Gap for Student Success Clear and effective communication is essential when addressing the needs of at-risk students.
To ensure they receive the support they need, establishing open lines of communication between students, parents/guardians, and teachers is paramount. Regularly scheduled meetings or conferences provide an opportunity for all parties involved to discuss concerns or progress openly.
Teachers should employ active listening skills when engaging with at-risk students. By creating a safe space where these individuals feel heard and understood without judgment or criticism, teachers can foster trust and rapport that encourages open dialogue about challenges or obstacles faced by the student.
In addition to teacher-student communication channels; involving parents or guardians in the support process is crucial. Sharing progress reports, discussing areas of improvement, and seeking input from parents/guardians ensures a collaborative approach that maximizes the student’s potential for success.
Evaluating the impact of interventions through ongoing monitoring
Subtitle: Tracking Progress Toward Triumph Implementing interventions without evaluating their effectiveness would be like navigating blindfolded. Continuous monitoring of the impact of interventions on at-risk students allows educators to make data-driven decisions and refine support strategies accordingly.
One common and effective method for ongoing monitoring is gathering regular feedback from students themselves. By administering surveys or conducting structured interviews, educators can gain insights into how well interventions are resonating with students and identify areas where adjustments may be needed.
This feedback loop not only empowers students by involving them in shaping their own educational journeys but also provides valuable information for educators to fine-tune their approach. Furthermore, analyzing academic performance data throughout the intervention process helps gauge progress and inform decision-making.
Tracking changes in grades, attendance records, or behavioral patterns allows educators to identify any emerging trends that may require additional support or modification of existing strategies. By providing personalized tutoring, implementing effective communication strategies with all stakeholders involved, and continuously monitoring the impact of interventions; we can give at-risk students every opportunity to succeed academically while fostering personal growth and development.
Benefits and Challenges in Implementing Learning Analytics
Improved Student Retention Rates and Academic Outcomes
Learning analytics and predictive modeling offer immense potential to improve student retention rates and enhance academic outcomes. By identifying at-risk students early on, educational institutions can provide targeted interventions and support tailored to individual needs.
This personalized approach allows educators to address specific challenges faced by struggling students, such as offering additional tutoring or mentoring programs. With the aid of learning analytics, teachers can better understand the factors contributing to student at-riskness and take proactive measures to prevent academic setbacks.
Furthermore, by leveraging data-driven insights from learning analytics, institutions can promote effective teaching practices that align with students’ learning preferences. This fosters a more engaging and inclusive learning environment.
The use of predictive models also enables educators to anticipate potential obstacles that students may encounter during their educational journey. This foreknowledge empowers teachers to make informed decisions about curriculum adjustments or instructional strategies that cater to diverse student needs, ultimately leading to improved academic outcomes.
Enhanced Decision-Making for Educators
Implementing learning analytics equips educators with powerful tools for evidence-based decision-making. By analyzing vast amounts of data collected from various sources, including student information systems and learning management systems, educators gain valuable insights into student performance trends, patterns, and behaviors. Armed with this knowledge, they can make informed decisions about the allocation of resources, curriculum design improvements, or targeted interventions for specific groups.
Additionally, learning analytics provides a comprehensive view of each student’s progress throughout their educational journey. This holistic understanding enables educators to personalize instruction methods based on individual strengths and weaknesses.
It also facilitates early identification of gifted students who may require advanced coursework or specialized enrichment activities. Overall, the integration of learning analytics into decision-making processes empowers educators with a data-driven approach that maximizes educational outcomes while fostering a supportive environment for all learners.
Conclusion
The use of learning analytics and predictive modeling in identifying at-risk students early on holds great promise for educational institutions worldwide. With the potential to improve student retention rates, enhance academic outcomes, and facilitate evidence-based decision-making for educators, these approaches are transforming education into a more personalized and effective experience. By harnessing the power of data, schools and universities can gain deep insights into student behaviors, needs, and challenges.
This valuable information enables proactive interventions that address individual circumstances and foster success. While challenges may arise in terms of data collection and privacy concerns, the benefits far outweigh the hurdles faced during implementation.
Embracing learning analytics is not only about optimizing educational processes but also about empowering students to overcome obstacles with personalized support. As we move forward in this digital age, let us embrace data-driven insights to unlock the full potential of every student, fostering a brighter future for all.